At the age of twenty weeks, a fetus is about the size of a bell pepper. “And the heart,” says Christian Baumgartner, as he forms a small gap between his thumb and index finger, “is only about as big as a cherry.” The comparison clearly illustrates why it’s difficult for physicians to locate and measure the tiny organs precisely during an ultrasound examination. They often need more time than anticipated to do it, and the tendency to make errors is considerable. Baumgartner experienced this himself with his youngest daughter. “During one of the examinations the doctor said her head was too large for her age,” he says. Later on, that turned out to be a mistake, but it was certainly hard on the nerves of the parents to be. That’s why the researcher from Switzerland is even happier that an ultrasound examination software he’s helped developing can in the meantime do it more precisely and easily. It’s already being used in an experimental setting in London, where doctors, who are involved in the research, can rely on the support of AI for prenatal ultrasound exams. The technology helps to locate the organs of the fetus and automatically measures them from an optimal angle.
Imaging techniques are supplying more and more complex data
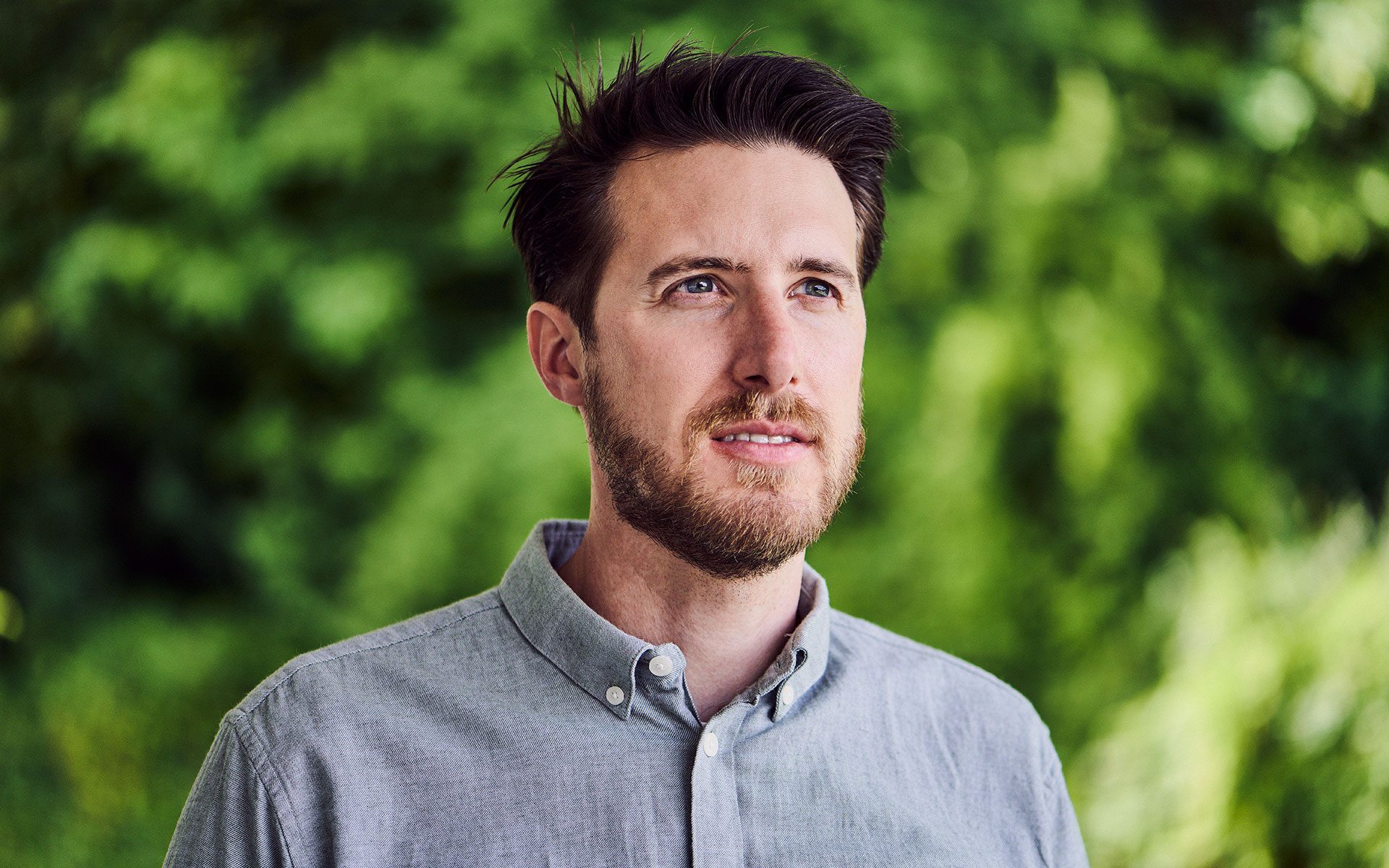
Christian Baumgartner leads the “Machine Learning in Medical Image Analysis” group at the Cluster of Excellence at the University of Tübingen © ELIA LUCA SCHMID/UNIVERSITY OF TÜBINGEN
That’s just one example of how artificial intelligence (AI) can change medicine. There are many more: Such as algorithms that can identify tumors on mammograms with astounding accuracy or determine reliably if an age spot is a harmless change in the skin or skin cancer. It’s no coincidence that machine learning (ML) is particularly prominent in medical image analysis. Whether it’s x-rays, ultrasound, computed tomography, positron emission tomography (PET), magnetic resonance imaging (MRI), or scintigraphy – modern medicine uses many procedures to look inside the body.
Many of them supply continually improving, increasingly detailed data, and as such, data that is ever more complex. Sometimes it’s a snapshot; while at others it’s over an interval of time, such as for analyzing a heartbeat or metabolic process. “Imaging techniques in medicine are delivering more and more and increasingly multidimensional data. On the one hand, that’s great, but on the other, it’s a substantial problem,” explains Baumgartner, who has been leading the “Machine Learning in Medical Image Analysis” group at the Cluster of Excellence at the University of Tübingen since February 2021. During daily routine at hospitals, time is scarce, as is the capacity to evaluate the data. There is already far more than doctors are able to analyze. From that perspective, AI will prove essential in order to efficiently utilize the flood of information. Beyond that, there’s an added value that the machines offer to medicine: They are more consistent than humans. “Put simply, algorithms don’t have bad days or get tired and unfocused when they’re doing overtime or haven’t had a coffee. Therefore, there are fewer human factors to distort results,” Baumgartner points out. What is more, continues the researcher, it’s normal for different radiologists and pathologists to come to different conclusions when they look at the same medical images or tissue samples. That’s a particularly big problem when it’s a matter of decisive questions, like “Is it cancer or not?” The answer to that determines the course of the patient’s treatment. “AI helps in such cases to get a clearer assessment and avoid misdiagnoses,” he adds.
Humans can learn from machines
Baumgartner first studied electrical engineering at ETH Zürich, before his initial encounter with medical image analysis while doing a Master’s in biomedical engineering. He found it so fascinating that he never looked back – not during his doctoral work at St. Thomas Hospital in London, nor while in his first post-doctoral job at Imperial College in London, nor during his second at ETH Zürich, and not while working for a year in private industry. The 37-year-old is also extremely happy he is now working in Tübingen on the transfer of new technologies into medical practice. Says Baumgartner, “It might sound kitschy, but I would really like my research to contribute to global progress. And because AI can undoubtedly, sustainably improve health care, I have a real chance to achieve that.”
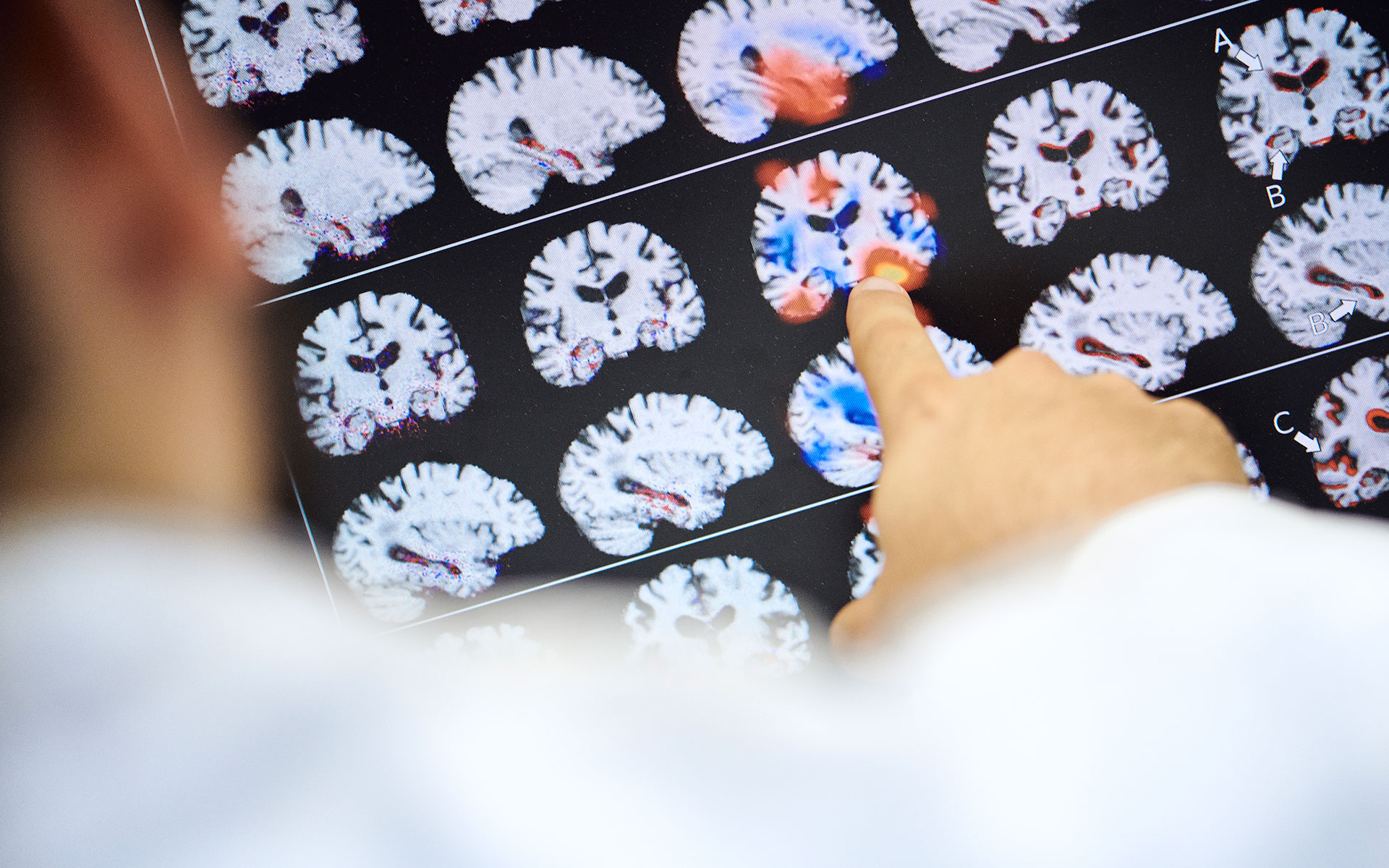
Modern medicine uses many procedures to look inside the body © ELIA LUCA SCHMID/UNIVERSITY OF TÜBINGEN
The researcher adds, that is all the more true, because algorithms won’t just improve existing procedures. They’ll also be able to contribute new knowledge and new diagnostic procedures to medicine. He says training algorithms and teaching machines things are just one aspect. The other is being able to learn something from the machines. That’s a point that really stimulates his interest in research. “Algorithms are, for example, capable of determining the age and sex of a person using a retinal image. They can also estimate their risk of heart attack or suffering from dementia. We don’t know precisely from which parameters the machines make that assessment. But it works reliably – and human doctors haven’t been able to do it up to now,” says Baumgartner, visibly excited as he clicks through a series of images on his computer screen. They show the retinal images he just mentioned – thin blood vessels tracing their way through an orange-red, round mass that’s a bit reminiscent of an egg yolk. So if machines in the meantime are able to do more than people, will they make doctors redundant in future?
Radiology will change fundamentally
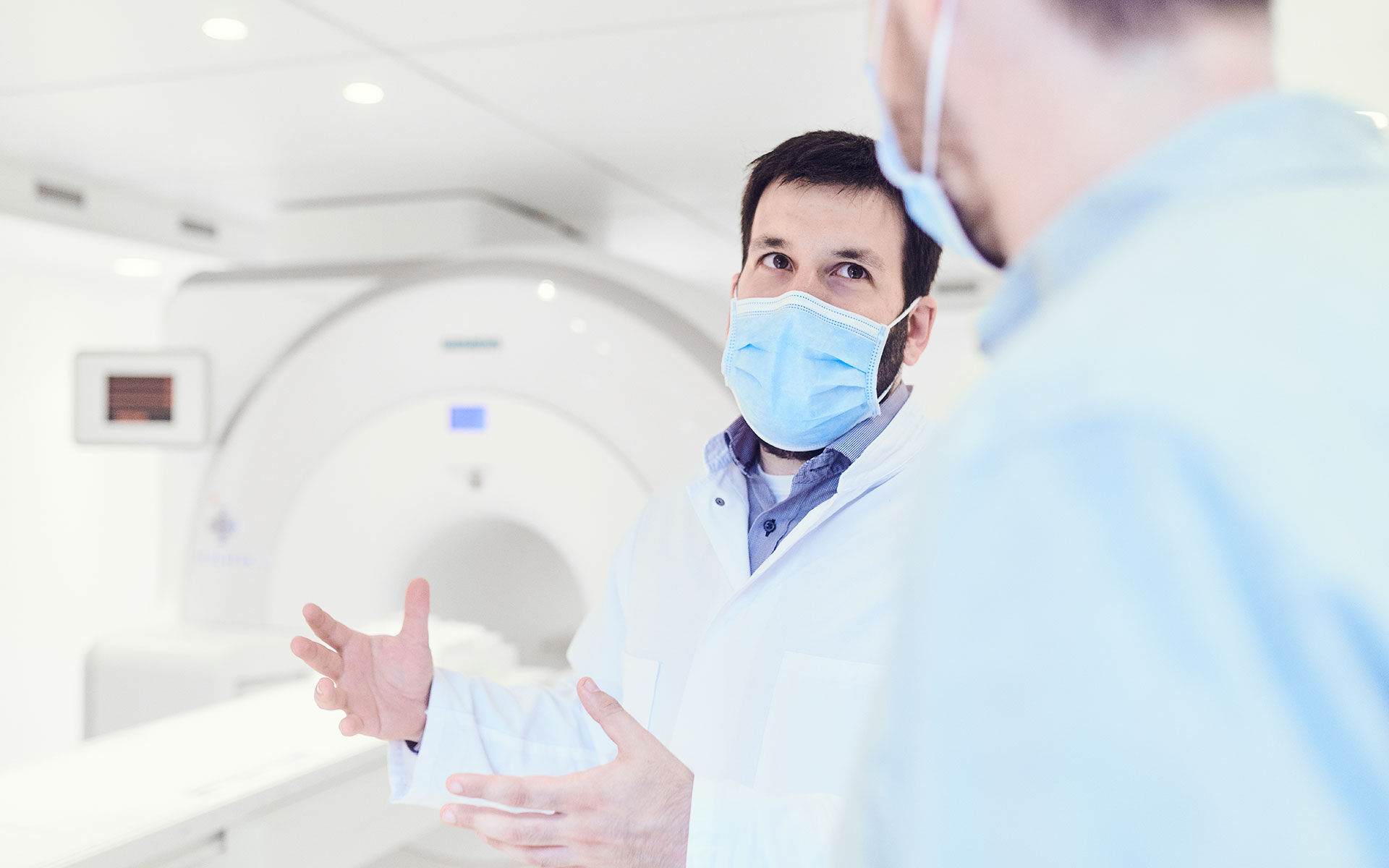
Professor Sergios Gatidis grins at the question. He’s a senior physician at Tübingen University Hospital, where his specialty is radiology. He is also a member of two clusters of excellence at the University of Tübingen. At the Cluster of Excellence called “iFIT” (“Image-guided and Functionally Instructed Tumor Therapies”), he’s working on oncological imaging. At the “Machine Learning” Cluster, he’s the expert for applying ML methods to medical imaging. He’s not afraid for his job. Says Gatidis, “I’m sure there will still be radiologists in the future. They’ll just work very differently to how they do today.” He expects that the specialty will fundamentally change during the next decade. Gatidis forecasts a shift so great that the discipline may need a new name – data medicine or diagnostic medicine, perhaps? But he says, for one thing, it’s still going to take some time; and for another, the advances won’t do doctors out of their jobs. “Instead of staring at images above all, we’ll choose the suitable procedure and algorithm, and let ourselves be assisted during the analysis and interpretation of the results, such as being able to filter out the images which require special medical attention from the continually growing mass of images,” says Gatidis.
He has no doubt the machines can beat people at simply recognizing human anomalies. “But that is by no means all there is to it,” he points out and illustrates with an example. A shadow on a lung, explains Gatidis, is just a shadow on a lung at first. Whether it’s a tumor, inflamed tissue, or something completely different has to be determined with the help of further information, such as laboratory results and tissue samples, and the patient’s medical history or current state of health. He says an infrastructure that automatically collates such data is a long way off. And although nobody is denying the potential of AI in medicine, he says it’s also true that, “Up to now, I’ve only seen a very few products that would pass the test of daily hospital use.”
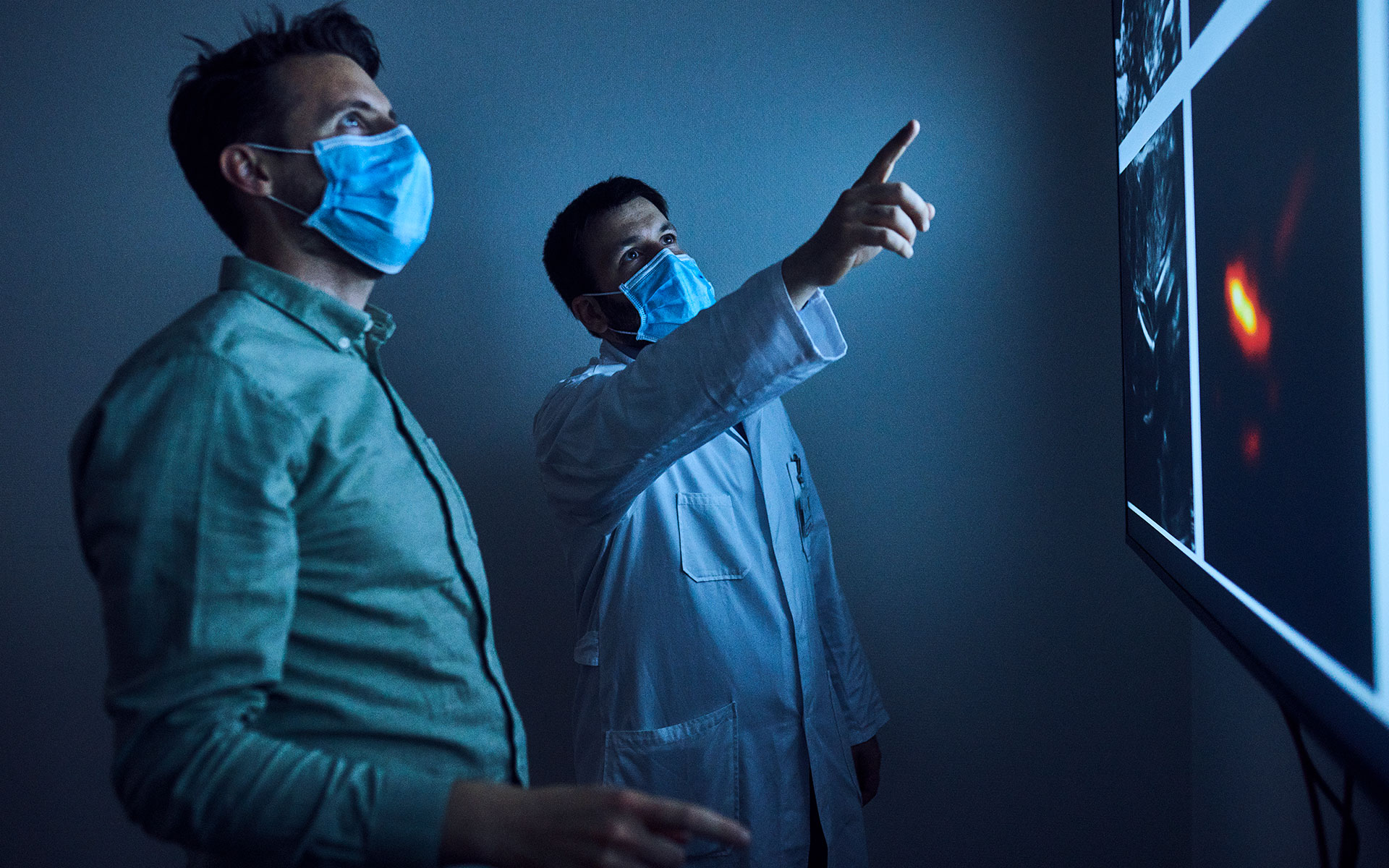
AI helps in such cases to get a clearer assessment and avoid misdiagnoses © ELIA LUCA SCHMID/UNIVERSITY OF TÜBINGEN
Actually, one of the biggest challenges is bringing the technology into practical use. That can only succeed if the products are simple and can be used intuitively, and if they actually add value, such as saving time or improving diagnoses. “They’ve got to make the lives of doctors noticeably easier from day one. Otherwise, no one will want to use them during hectic, daily hospital routines where there’s already plenty of work,” says Gatidis. One success story is the automatic measuring of organ volume, explains the radiologist, such as the determination of the size of the chambers of the heart during a heartbeat. A radiologist, he says, would need a half hour to do that, while the machine does it in less than a minute. “You don’t think twice about that,” says Gatidis, while adding that such examples are currently still rare.
Enormous amounts of data can benefit individuals
Christian Baumgartner knows that, too. Collaborating with actual medical users of the technology is extremely important to him. “The best technologies won’t help a thing if we overlook reality in our thinking,” he says. Baumgartner is often coming back to the interface between research and practice, but says he finds, “Science alone can’t revolutionize healthcare. It takes companies that apply the input and make it into practicable, comfortably useable products.” That’s happening right now, for example, in Britain, he says, where algorithms recently began being used to support breast cancer screenings in a major way. In addition to the technology transfer, Baumgartner is also interested in the philosophical and psychological questions raised by technological research. How can humans and machines collaborate well? What happens, for instance, when the doctor and the algorithm come up with different results? Or the doctor or patient are unable to understand the diagnosis of AI? “Shaping the cooperation will be enormously exciting,” says the researcher.
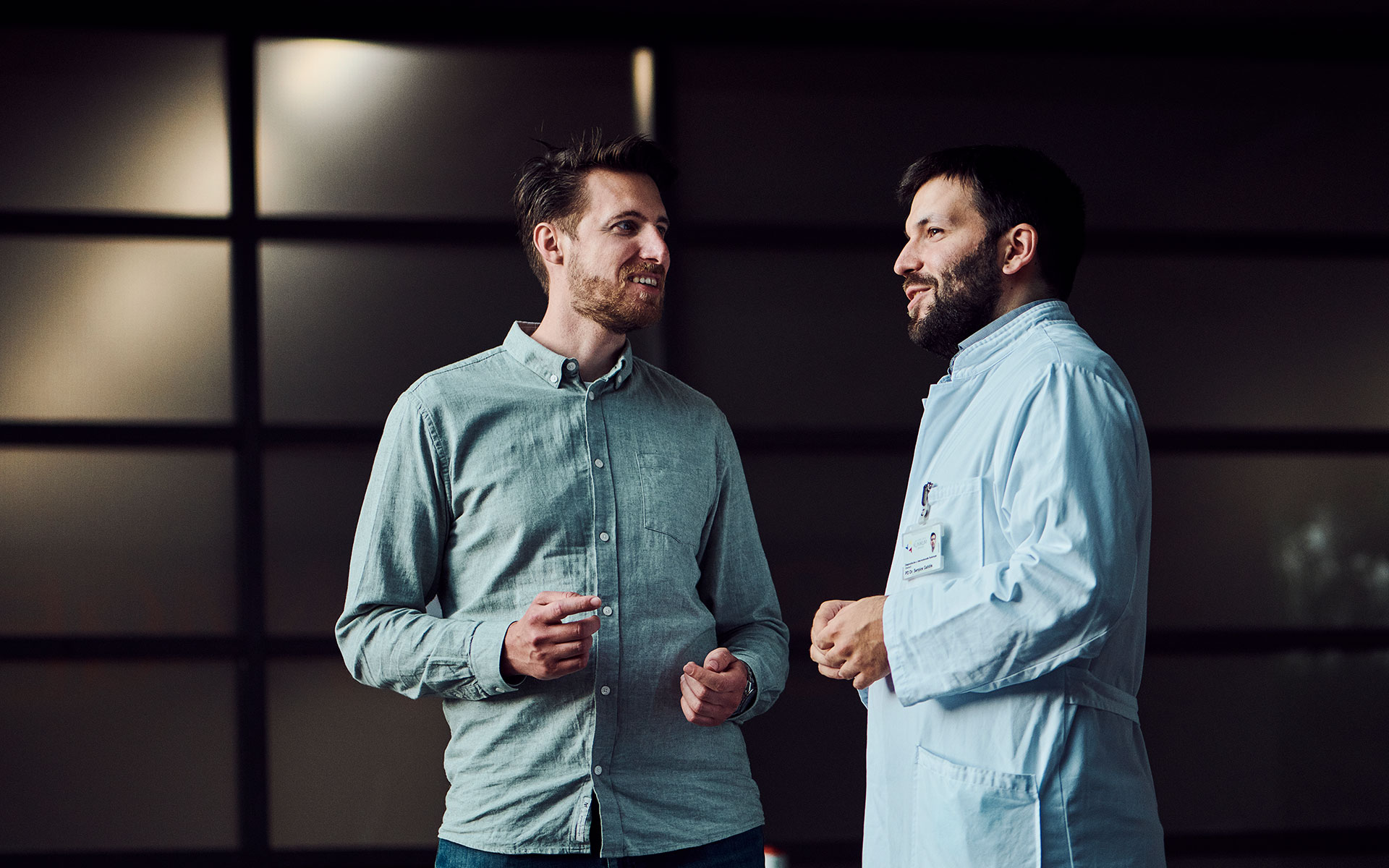
Baumgartner and Gatidis work interdisciplinarily at the Cluster of Excellence “Machine Learning” © ELIA LUCA SCHMID/UNIVERSITY OF TÜBINGEN
Cooperation. This key word is important to Baumgartner. Human against machine? He won’t touch that narrative. “There are areas where machines are superior to people. And others, in which machines can’t do what people do. To ensure the patient profits, both have to be incorporated,” says Baumgartner. He and his colleague Gatidis both have no doubt that can be done successfully and ML will revolutionize healthcare to a degree. Not least because it’s being driven by the megatrend of personalized medicine. At first it seems like a paradox that enormous amounts of data will ultimately benefit individuals, yet Baumgartner explains, “The recognition of patterns actually helps in making individual decisions about treatment.”
When will the technology become incorporated into daily hospital routine? Baumgartner hesitates, “Within the next ten years, AI will already have a much greater influence on medicine than it does today.” After all, looking into the future is ultimately even more difficult than looking into the body’s interior.
Text: Nina Himmer
Christian Baumgartner was a member of our cluster and head of the independent research group “Machine Learning in Medical Image Analysis” from 2021 to 2024. Currently, he is assistant professor for Health Data Science at the University of Lucerne, Switzerland.
Sergios Gatidis was a member of our cluster from 2020 to 2023 and is currently associate professor for radiology at Stanford Health Care, USA.
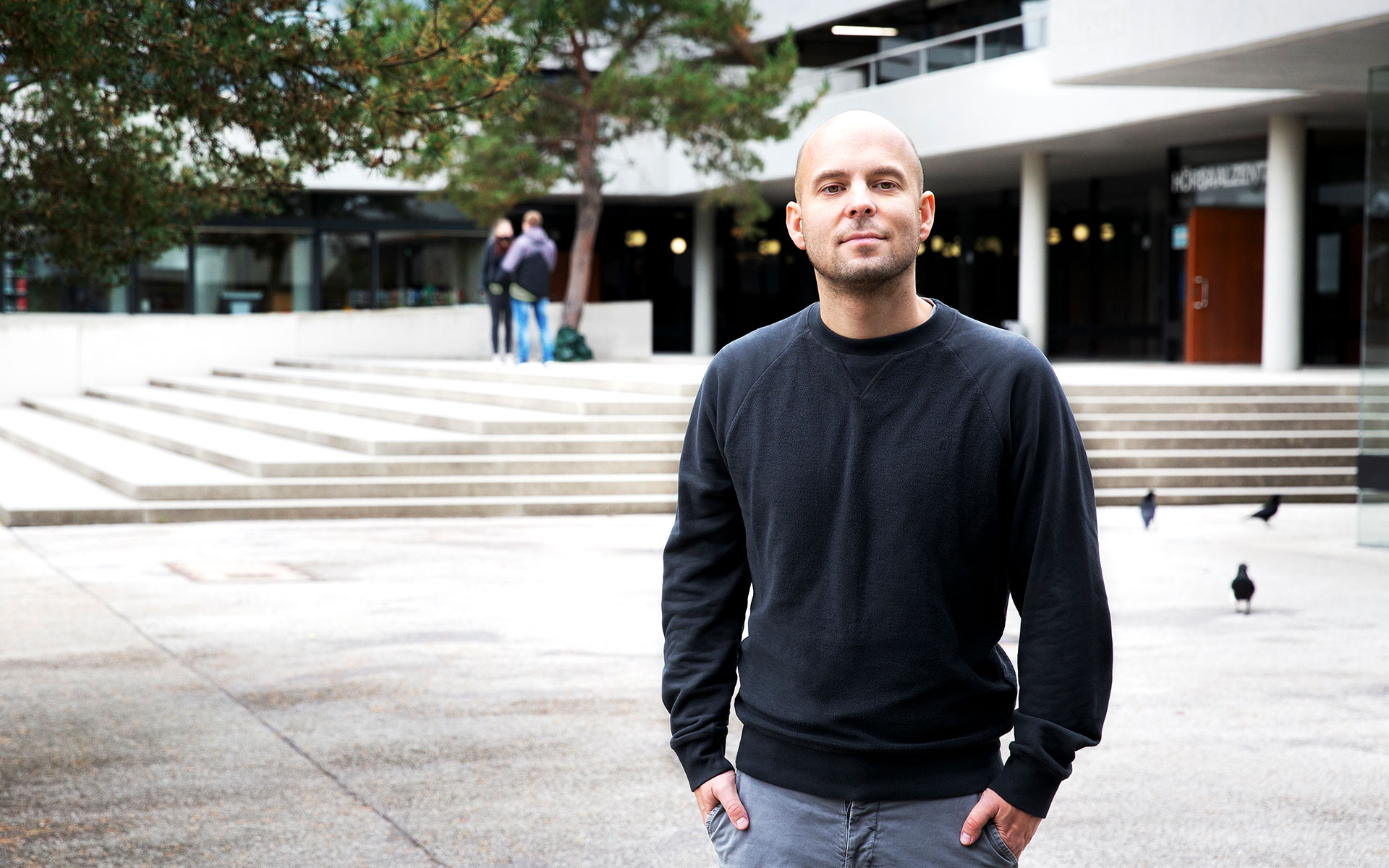
Where Algorithms and People Are Allies
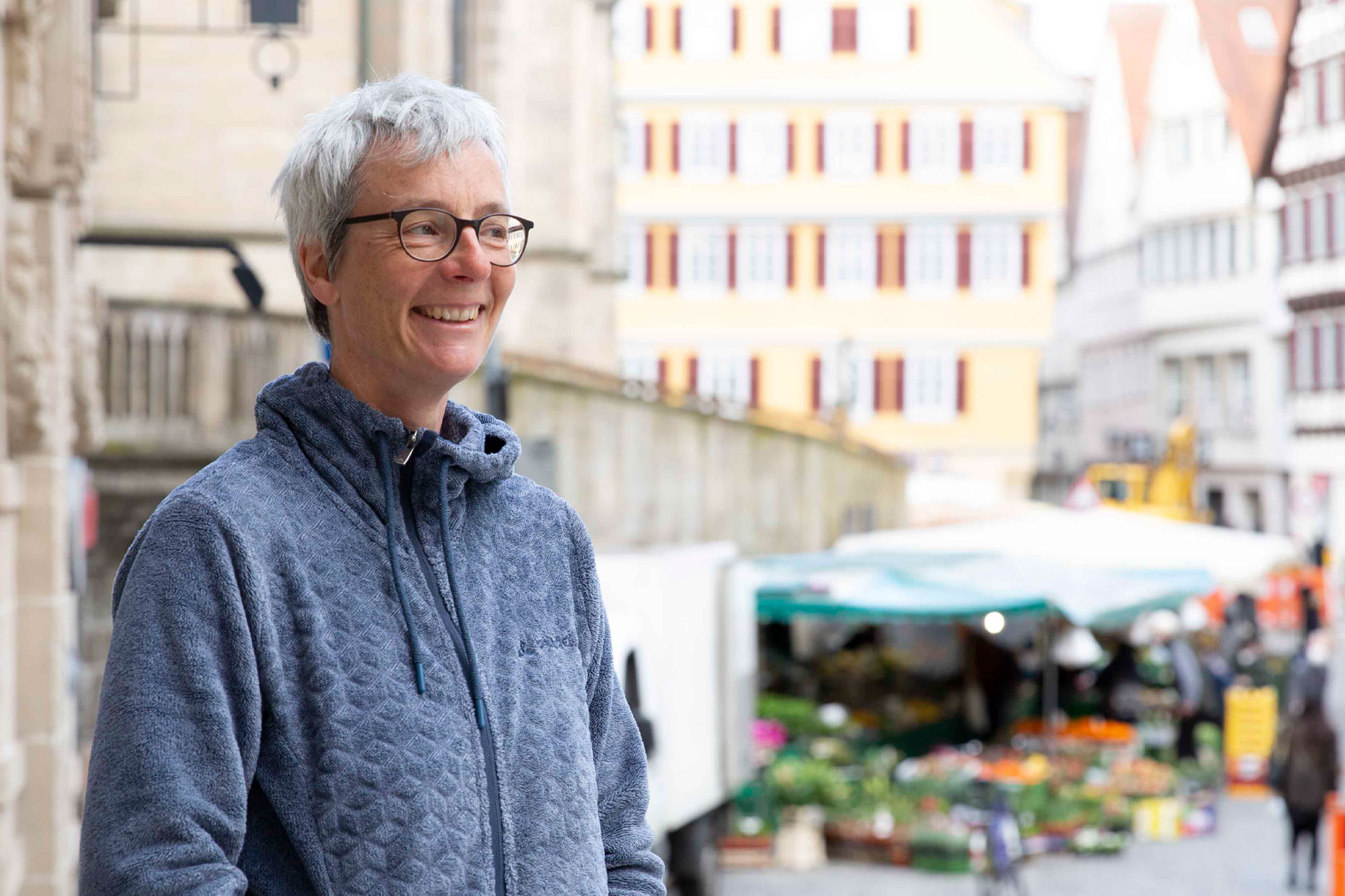